A Statistical Framework and the Problem of Disengaged Survey Responders
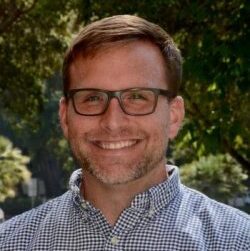
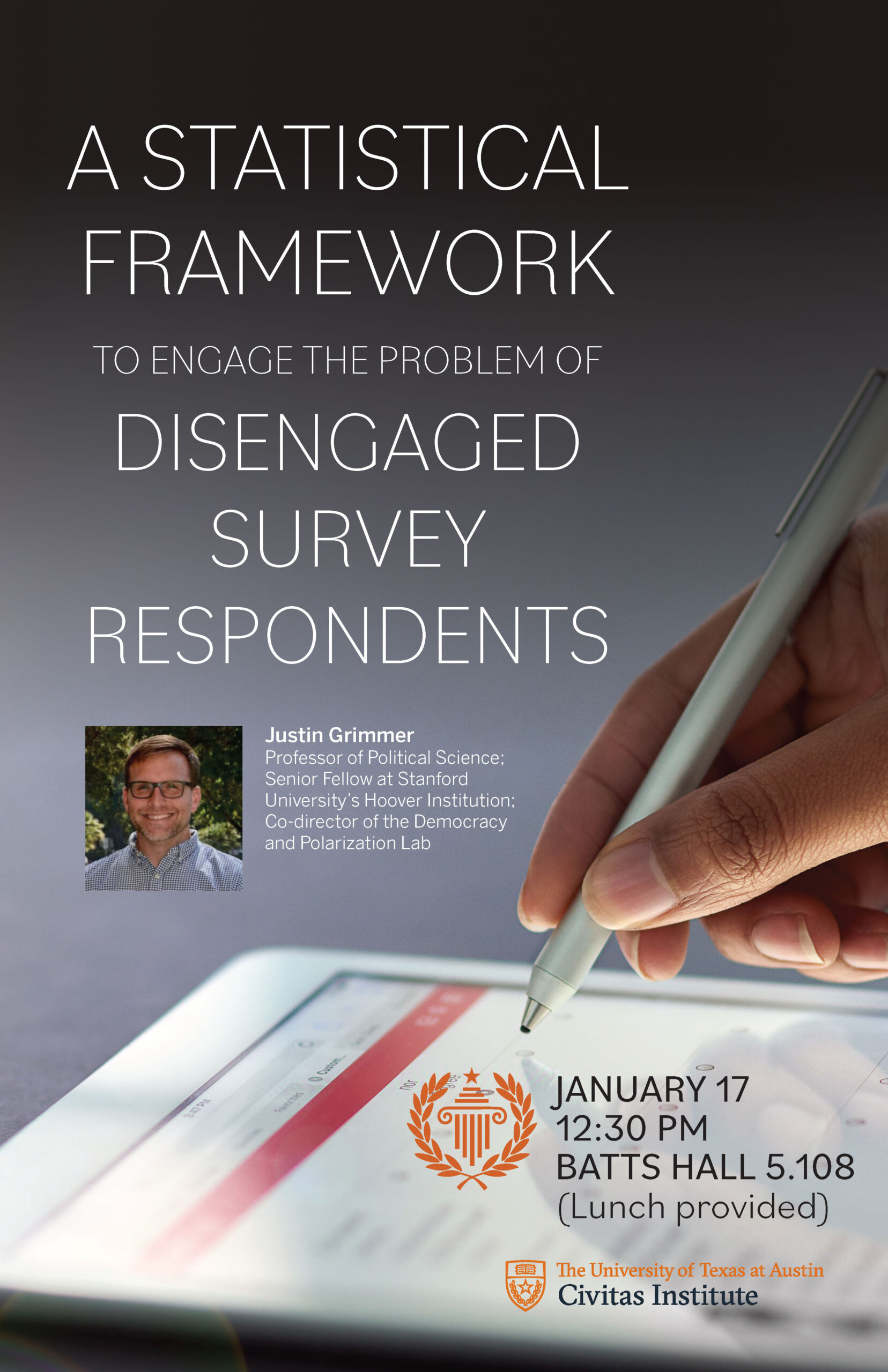
Researchers in academia, government, and industry increasingly rely upon online surveys to measure public opinion and to assess the effect of interventions because of their lower cost than over the phone and in-person surveys. But with their lower cost online surveys increase the risk of bias from disengaged respondents–a bias that remains even after firms and researchers use well-developed filters to capture disengaged respondents. We introduce and apply a statistical framework to show that the standard practice of dropping respondents who fail an attention check implicitly changes the population studied—from the overall population to the population who pass the attention checks. Using our framework we introduce a set of assumptions sufficient to partially and point identify the overall population’s attitudes and treatment effects from surveys polluted by disengaged respondents. We show how our assumptions can be applied to flexibly estimate average opinion and treatment effects, extending popular causal inference tools such as Double Machine Learning. We demonstrate our framework and proposed estimators to estimate support in the population for political violence after an election and the extent of polarization in the public.
Lunch will be provided. Please RSVP at: https://bit.ly/GrimmerLecture
Justin Grimmer is a Professor of Political Science and a Senior Fellow at the Hoover Institution at Stanford University. He co-directs with the Democracy and Polarization Lab. His work examines how representation occurs in American democracy and methods to improve its practice, while also developing and using new statistical and machine learning tools. Along with Molly Roberts and Brandon Stewart he is the author of the recently released book “Text as Data: A New Framework for Machine Learning and the Social Sciences”.